Companies use Machine Learning to make their processes smooth, fast, and convenient. However, there are barriers to machine learning, and the journey to adopting these kind of projects can be challenging.
The process can be overwhelming and can stir confusion and doubt about its efficiency. This is due to the challenges businesses encounter along the way, which slow down their goals in growing the business.
But all of these hurdles come with a solution, so with a little effort into making processes suitable and ensuring all aspects of Machine Learning are given focus, this endeavor can be rewarding.
These are the three most common obstacles to machine learning your business may encounter and how you can overcome them.
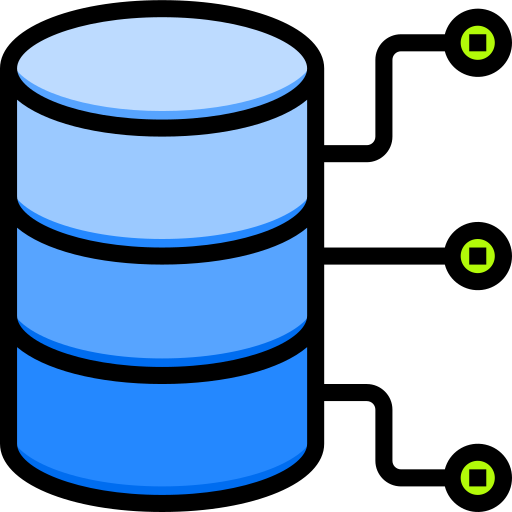
Inefficient Data and poor security
One of the first barriers to Machine learning in businesses that use try to adopt these technologies is that they often often choose to save vast amounts of data in different storages, making the process longer and more time-consuming.
The extraction of data is another thing to consider. Data security is crucial in machine learning projects, given that breach is possible, specifically for sensitive data containing personal information.
Data encryption may be done before the model comes in, allowing a more challenging data access process.
To overcome this hurdle, the separation of sensitive data is needed. This option also minimizes the risks in security.
Lesser sensitive data should be more accessible by reliable team members so that when it is needed, it can be used as soon as possible without waiting time.
Accessibility to data also makes processes faster and more convenient when it comes to development, testing, and collaborating with others.
Additionally, understand that machine learning models are only sometimes straight out of perfection. It still has flaws, makes mistakes, and is always open for changes as you go through your projects.
Data access and security must come hand in hand in resolving this aspect of machine learning. By ensuring flexible yet firm accessibility and security, you can freely work with your projects without thinking of data breaches.
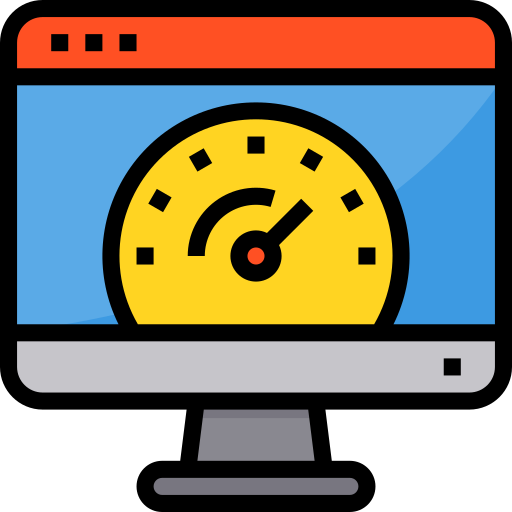
Lack of robust test and experimental phases
Our second of the three barriers to machine learning is that businesses need to understand that machine learning technology must undergo testing and experimentation just like in every other system.
These phases are essential for having good data. It also serves as the benchmark of your machine learning project. There is no room for incomplete data and dirty and noisy data if you want your machine learning project to thrive.
As artificial intelligence may not always be flawless from the get-go, testing allows comparison to the accurate data.
While some businesses tend to test it in a mock process, the key to achieving the right results is injecting the machine learning model into your actual strategy.
Companies may prefer something other than this option, especially for those new to digitalization. For large scale projects, it can be difficult to apply such trials right to the real process because of fear of shaking the status quo.
A solution for this concern is to strengthen the department where experimentation phases occur. A strong support system that can serve as a backup or safety net should be in place for each test, specifically if it involves large-scale machine learning technologies.
It is essential to build independent sandboxes, allowing various teams to experiment on their own using different approaches.
Using various solutions and methods, each experiment is tested up to its maximum, with results showing what it can offer. All results from each test will reveal what deserves to go up in the next phase or what needs to be considered for final use.
For example, suppose you are to adopt a machine vision AI for your company. In that case, it is necessary that tests include visual inspection, positioning, defect detection, identification, sorting, and tracking using different methodologies.
The best result will likely be your ultimate choice, with chances of getting improved, revised, or scrapped later.
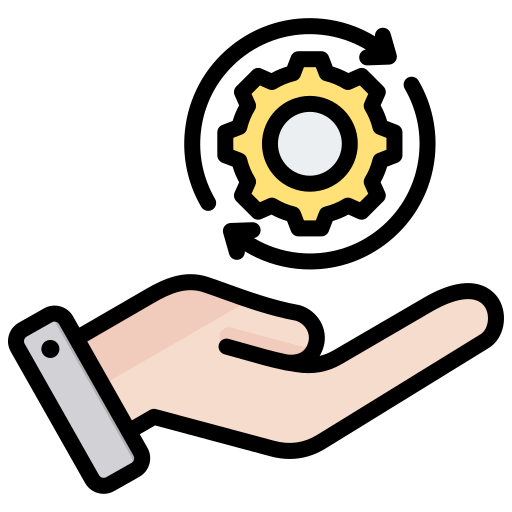
Inability of business processes to adapt to changes
Gone are the days when firm business processes were the norm in digital transformation. Companies that likely succeed in machine learning welcome new data with flexibility and openness to what comes next.
Machine learning demands continuous evolution in business processes, starting with businesses’ capacity to adapt to rapid changes, evolving skill sets, and diverse growth opportunities.
Also included in the need for flexibility are data governance, data integration, and data exploration, all of which require crucial supervision and monitoring. These processes ensure all data are evaluated and all scopes are checked, tested, and assessed.
One aspect to think of under this challenge is inadequate machine learning infrastructure. When adapting this technology, companies must understand why upgrading infrastructure with flexible storage and hardware acceleration is vital to churn considerable amounts of data.
The lack of such competitive facilities defeats the purpose of innovation and can slow down machine learning opportunities.
Moreover, machine learning technology is bound to break down processes and regulations and does not stop with limitations.
One thing’s for sure, machine learning does not promise quick success.
With machine learning, businesses are required to adopt a culture of agility and tenacity. By being agile, businesses learn from failed tests and move on quickly to study the new ones.
This solution lets companies deal with failure fast, recognize the opportunities for learning, and veer away from spending too much capital on processes that no longer work.
It is a waste of time in machine learning and in adopting artificial intelligence in general if companies allow themselves to be dragged by failures from its past projects.
By quickly acting after failure, companies reach the goal of building a solid machine learning strategy ideal to withstand changes.
Machine learning is a deep learning technique that provides many windows for improving your business process.
You may encounter hurdles as you develop your machine learning projects, and they are inevitable.
While it has imperfect models, algorithms trained on data sets, and many inaccuracies that come with it, it does not mean that they are unreliable. You can learn a thing or two from each model and create a better version for your next.
These challenges to machine learning may seem overwhelming for some businesses, but overcoming them opens more opportunities for them to grow.
- Marr, Bernard (Author)
- English (Publication Language)
- 272 Pages - 10/26/2021 (Publication Date) - Kogan Page (Publisher)
Summary on the main barriers to Machine Learning
In this post we have covered some of the main barriers to machine learning.
Despite their perceived difficulty, these obstacles can be tackled by clearly aligning the companies goals towards becoming more data driven, incorporating security standards, and driving change management and an innovation mentality within the organization.
We hope that this article on the obstacles to machine learning was useful, have a wonderful day, and as always, thank you for reading How to Learn Machine Learning!
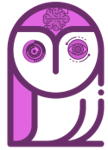
Subscribe to our awesome newsletter to get the best content on your journey to learn Machine Learning, including some exclusive free goodies!